AI Governance Framework: The CEO’s Survival Guide
AI is changing how businesses operate today. But without proper oversight, it can cause more harm than good. A resume screening system might automatically reject candidates with employment gaps, unfairly penalizing parents returning to work. I’ve worked with businesses that fully trusted AI, only to realize too late that it needed guardrails. The problem wasn’t AI itself—it was the lack of implementing a clear AI Governance Framework.
Businesses rush to adopt AI, but few put governance first. That’s a problem. A 2023 McKinsey report found that only 21% of companies have clear AI risk management practices. That means most organizations have no safeguards in place when AI goes off track.
AI governance isn’t about adding complexity. It’s about setting rules, assigning responsibility, and ensuring AI supports business goals without unintended consequences. Governance prevents legal issues, keeps AI aligned with ethical standards, and improves accountability. It also builds trust with customers, employees, and regulators.
Where do you start? The first step is defining policies—what AI can and cannot do in your business. Next, establish oversight—who monitors compliance, handles risks, and makes the final call. Regular audits keep AI in check, while transparency helps users understand how decisions are made.
AI shouldn’t be a mystery. With the right governance, businesses stay in control. This guide lays out the essential steps to building a governance framework that keeps AI reliable, compliant, and aligned with business goals.
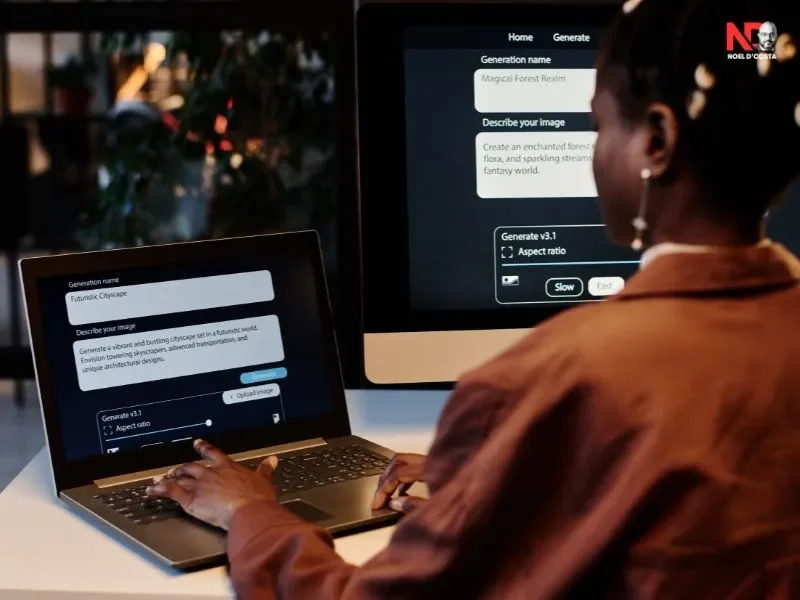
Key Regulations Related to AI
With so many AI tools appearing everywhere, it has become to assess compliance to data privacy. Governments worldwide are cracking down, and businesses that ignore compliance are paying the price.
In 2023, the FTC fined Amazon $25 million for mishandling children’s voice data in Alexa recordings. AI regulations aren’t “coming”—they’re already here.
The EU AI Act is one of the strictest frameworks today. It classifies AI by risk level—low, high, and unacceptable. High-risk AI, like facial recognition and credit scoring, faces strict transparency and oversight rules. Companies in sensitive industries must document decisions, test for bias, and involve human oversight.
Privacy laws like GDPR and CCPA put limits on AI-driven data collection. AI must get consent, explain its decisions, and let users opt out. In 2021, WhatsApp was fined $267 million under GDPR for not informing users how their data was used.
We know that the U.S. AI Bill of Rights isn’t legally binding, but federal agencies are already enforcing AI bias rules. The EEOC is cracking down on AI-driven hiring discrimination. If your company uses AI for recruitment, auditing for bias is now mandatory.
ISO 42001, the first AI governance standard, gives businesses a roadmap for risk assessment and compliance monitoring.
Regulations are evolving fast. Companies that document AI decisions, address bias, and stay compliant will build trust and won’t pay fines.
The question isn’t if AI laws will impact your business. It’s when.
Key Regulations Related to AI
Regulation | Jurisdiction | Key Requirements | Enforcement Authority |
---|---|---|---|
EU AI Act | European Union |
|
European Commission |
GDPR | European Union |
|
Data Protection Authorities (DPA) |
CCPA | United States (California) |
|
California Attorney General |
ISO 42001 | Global |
|
ISO (International Organization for Standardization) |
AI Bill of Rights | United States |
|
White House Office of Science and Technology Policy |
Produced by Noel D'Costa | Visit my website: https://honeydew-sheep-964865.hostingersite.com
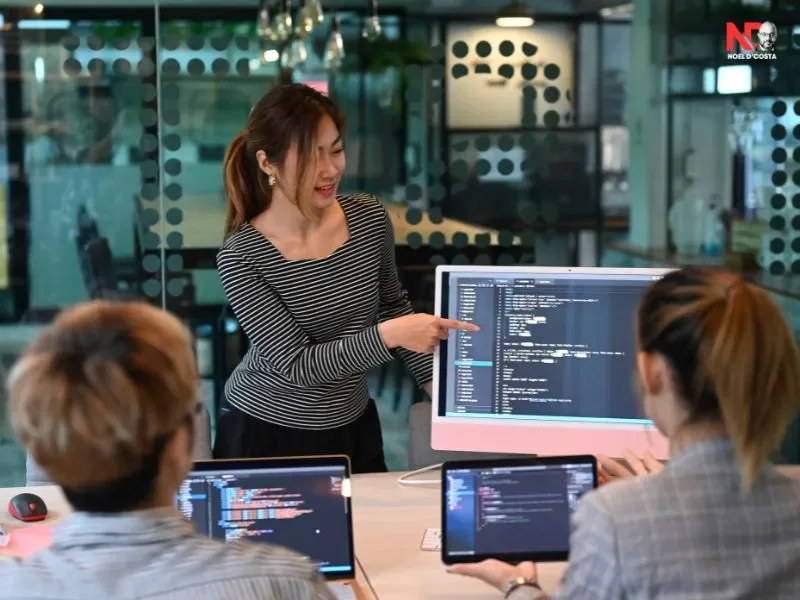
Key Components of an AI Governance Framework
AI is making real decisions that impact real people. A hiring tool rejects qualified candidates. A fraud detection system wrongly flags transactions. A chatbot gives misleading advice.
Without governance, these problems multiply. Companies that don’t manage AI risks face lawsuits, lost trust, and compliance failures.
1. AI Ethics & Responsible AI Principles
AI shouldn’t be implemented without proper controls. Companies need clear ethical guidelines to prevent bias, discrimination, and shady practices.
- Define acceptable AI use cases—Know where AI belongs and where it doesn’t.
- Set policies that prevent bias—Don’t let AI make discriminatory or unethical decisions.
- Ensure AI decisions are explainable—If someone questions an AI-driven outcome, there should be a clear answer.
2. Risk Management & Compliance
Regulators aren’t messing around. The EU AI Act, GDPR, and ISO 42001 demand AI accountability. Companies that ignore compliance risk massive fines.
- Know which AI applications need regulatory approval.
- Assign a compliance officer—Someone should track AI-related legal changes.
- Run regular audits to make sure AI models follow legal and ethical standards.
3. AI Transparency & Explainability
AI shouldn’t be a black box. If a system denies a loan or rejects an insurance claim, people deserve to know why.
- Use explainable AI models—Make decision-making clear and logical.
- Provide human-readable reports for employees and customers.
- Train staff to interpret AI decisions instead of blindly trusting them.
4. Bias & Fairness in AI Models
AI can reinforce discrimination if left unchecked. A 2018 MIT study found facial recognition misidentified dark-skinned women 34% of the time.
- Test AI models for bias across race, gender, and socioeconomic factors.
- Retrain AI regularly using diverse and representative datasets.
- Require human review for high-risk AI decisions.
5. Data Security & Privacy Measures
AI handles sensitive data. A security failure can expose financial, health, and personal information.
- Encrypt AI-generated data and restrict access.
- Follow global privacy laws—GDPR, CCPA, and beyond.
- Implement retention and deletion policies to prevent misuse.
6. Human Oversight & Decision Accountability
AI should assist, not replace, humans. When AI makes big decisions, a human should have the final say.
- Assign human reviewers to oversee AI decisions.
- Create escalation pathways for AI mistakes.
- Require regular manual audits of automated decisions.
AI without governance is a liability. But with the right framework, it becomes a competitive advantage. The companies that get this right will stay ahead of legal risks and build trust.
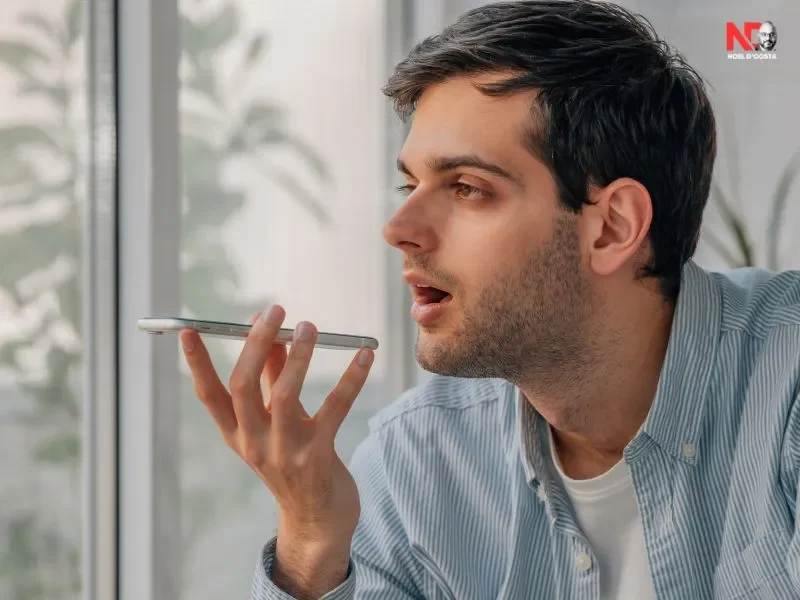
How to Develop an AI Governance Framework
AI can’t be left to run unchecked. Without clear guidelines, it can make decisions that put your business at risk. Compliance violations, bias, security gaps—these aren’t just theoretical risks.
I’ve seen companies struggle because they didn’t put the right governance in place early. You don’t want to be in that position. A governance framework ensures AI stays ethical, transparent, and aligned with your business goals.
Step 1: Define AI Governance Goals
Every business needs a clear purpose for AI. What should it achieve? What risks should it avoid? If you don’t set these goals upfront, AI can take your business in a direction you never intended.
- Identify AI applications that affect compliance, security, or customer trust.
- Set measurable goals for accuracy, fairness, and accountability.
Step 2: Establish Policies & Standards
AI needs rules. Without them, teams work in silos, and governance falls apart. I’ve worked with businesses that rushed AI into production without clear policies, and fixing that later was painful. You don’t want to go down that road.
- Draft clear internal guidelines on AI development and risk assessment.
- Ensure compliance with GDPR, CCPA, ISO 42001, and industry-specific regulations.
Step 3: Assign AI Governance Roles
Someone needs to own AI oversight. If nobody is accountable, AI mistakes get ignored until they become serious problems.
- Form an AI ethics board or compliance team.
- Assign roles for AI auditing, legal reviews, and risk management.
Step 4: Implement Risk Management Protocols
AI models drift. What works today might fail tomorrow. If you’re not continuously monitoring AI, small errors turn into big liabilities.
- Monitor AI performance, fairness, and security vulnerabilities.
- Set thresholds for retraining or decommissioning underperforming models.
Step 5: Ensure AI Transparency & Explainability
AI decisions shouldn’t be a mystery. If you don’t know how AI is making decisions, how can you trust it?
- Document how AI models work and how they make decisions.
- Provide explanations to regulators, employees, and affected users.
Step 6: Conduct Continuous Audits & Improvements
AI governance isn’t a one-time project. You and I both know technology evolves fast, and regulations will keep changing. If you don’t review and update your governance framework, you’ll fall behind.
- Schedule periodic compliance reviews and risk assessments.
- Adjust AI strategies based on real-world performance and new regulations.
AI is powerful, but it needs guardrails. If you put the right governance in place, you’ll stay in control. If you ignore it, AI will control you.
How to Develop an AI Governance Framework
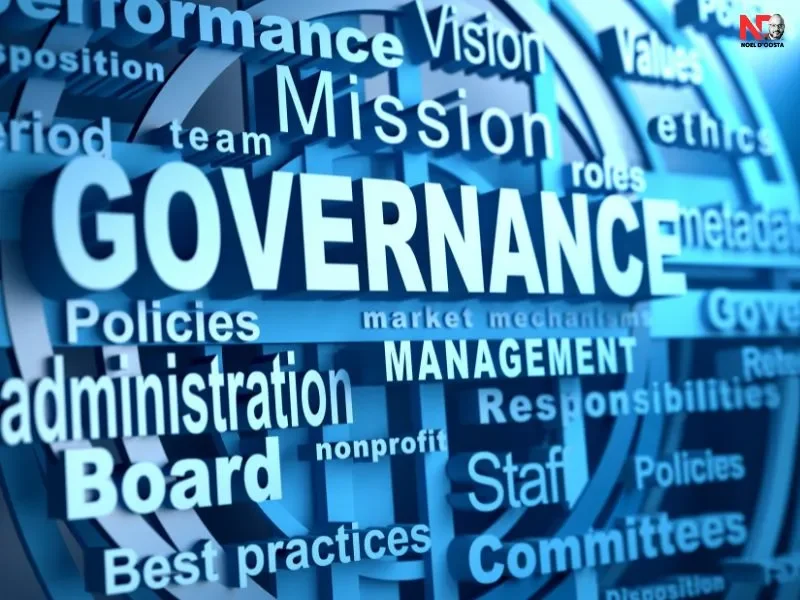
Best Practices for AI Governance Implementation
AI governance isn’t just a compliance task. It’s about making AI work for your business while avoiding costly failures. Companies that ignore governance face reputational damage, legal trouble, and financial losses.
- Microsoft pulled its AI chatbot Tay from Twitter in less than 24 hours after it started spouting offensive content.
- Google’s facial recognition AI misidentified people of color, leading to widespread backlash.
- Amazon scrapped its AI hiring tool after it was found to discriminate against women.
These are examples of avoidable business risks.
Embedding AI Governance into Risk Management
AI doesn’t operate in a vacuum. It impacts finance, security, HR, and customer trust. That’s why AI governance must be part of Enterprise Risk Management (ERM).
- Identify high-risk AI applications → AI used in hiring, lending, or healthcare needs stricter oversight than a chatbot answering FAQs.
- Establish accountability → AI shouldn’t make unchecked decisions. Compliance teams, risk managers, and data scientists must oversee AI operations.
- Monitor AI models in real-time → What worked six months ago may be broken today. Track performance and flag risks early.
- Audit AI like financial records → If AI influences high-stakes business decisions, regulators will demand transparency.
AI Compliance Frameworks: What You Need to Follow
AI regulations aren’t on the horizon—they’re here. Companies that fail to align risk lawsuits and massive fines.
- NIST AI Risk Management Framework → Helps businesses develop trustworthy AI.
- OECD AI Principles → Focuses on fairness, transparency, and accountability.
- ISO 42001 → The first structured AI governance standard.
- EU AI Act & GDPR → Strictest AI laws globally—ignoring them isn’t an option.
Companies that don’t follow these risk privacy violations, biased AI, and lost consumer trust. Those that do have a roadmap for responsible AI and that is an AI Risk Management Framework.
Lessons from Google & Microsoft: How Big Tech Fixed AI Failures
Even the world’s biggest tech firms learned AI governance the hard way.
- Google created an AI Ethics Board to review high-risk AI projects before launch.
- Microsoft built Responsible AI Guidelines, requiring impact assessments for AI tools.
- Both companies now use bias detection tools—Microsoft even built Fairlearn, an open-source toolkit to reduce AI bias.
These changes weren’t preemptive. They were made after AI failures hit the headlines. Companies that get governance right from the start avoid these costly mistakes.
AI Governance Is a Business Imperative
The choice isn’t whether to implement AI governance—it’s whether you’ll do it before or after disaster strikes.
Businesses that take AI risk seriously will gain trust, avoid lawsuits, and prevent financial loss. Those that don’t will spend time reacting to AI failures instead of preventing them.
Is your AI governance strategy in place? If not, now’s the time to fix it.
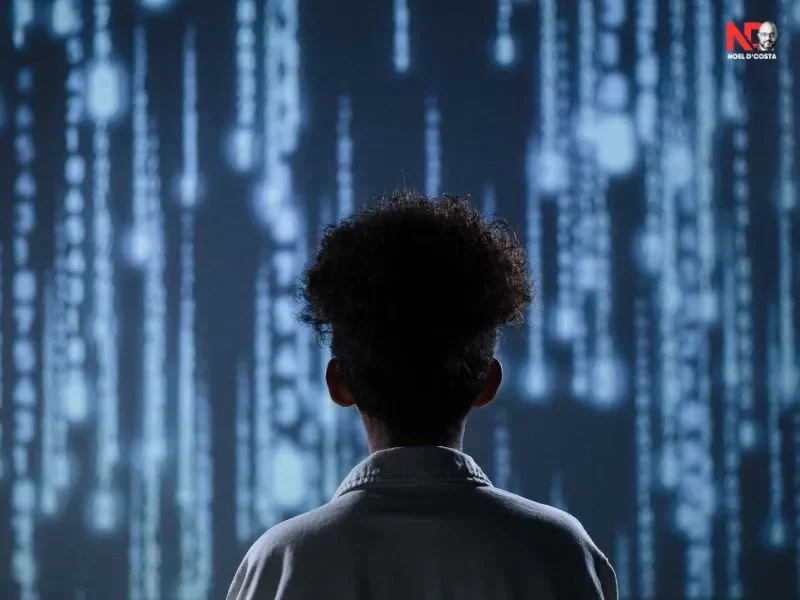
Challenges & Solutions in AI Governance
In my opinion, AI governance is an ongoing challenge. Regulations shift, bias creeps in, and businesses struggle to scale oversight. Companies that fail to address these issues early risk compliance fines, reputational damage, and unreliable AI models. So how do you keep AI accountable, fair, and scalable? It starts with tackling four key challenges.
Regulatory Uncertainty → Staying Updated on AI Laws
AI laws are evolving fast. The EU AI Act introduces risk-based regulations, while GDPR already enforces data protection. The U.S. AI Bill of Rights is pushing for transparency. If you’re not tracking these changes, compliance gaps will surface.
- Solution: Assign legal and compliance teams to monitor global AI regulations.
- Solution: Implement AI policy tracking tools to stay ahead of new requirements.
- Solution: Conduct regular legal audits to ensure AI models meet new standards.
AI Bias & Ethical Concerns → Implementing Fairness Checks
Bias isn’t a theoretical issue—it’s real. A 2019 study found that Amazon’s AI hiring system favored men over women due to biased training data. Left unchecked, bias leads to discrimination and legal risks.
- Solution: Run bias audits on AI models before deployment.
- Solution: Use fairness testing tools like IBM’s AI Fairness 360 or Fairlearn.
- Solution: Diversify training data to improve AI decision-making across demographics.
Scalability of AI Governance → Automating Compliance Monitoring
Manually reviewing every AI model isn’t sustainable. AI governance must scale with automation.
- Solution: Deploy automated compliance dashboards to track AI decision accuracy.
- Solution: Set up real-time alerts when AI models drift from expected performance.
- Solution: Integrate governance tools that log AI decisions for audit trails.
Lack of AI Expertise → Training Employees on AI Policies
AI governance isn’t just an IT issue—it requires company-wide awareness. A survey by Gartner found that 56% of organizations lack AI expertise. If employees don’t understand AI risks, governance won’t stick.
- Solution: Create AI governance training for non-technical teams.
- Solution: Develop internal guidelines outlining ethical AI use.
- Solution: Appoint AI governance leads to oversee compliance across departments.
AI governance won’t fix itself. The businesses that invest in risk management, fairness, and compliance automation will stay ahead—those that don’t will face legal, financial, and operational setbacks.
Challenges & Solutions in AI Governance
Challenge | Description | Solutions |
---|---|---|
Regulatory Uncertainty | AI laws are evolving fast. The EU AI Act introduces risk-based regulations, while GDPR already enforces data protection. The U.S. AI Bill of Rights is pushing for transparency. If you’re not tracking these changes, compliance gaps will surface. |
|
AI Bias & Ethical Concerns | Bias isn’t a theoretical issue—it’s real. A 2019 study found that Amazon’s AI hiring system favored men over women due to biased training data. Left unchecked, bias leads to discrimination and legal risks. |
|
Scalability of AI Governance | Manually reviewing every AI model isn’t sustainable. AI governance must scale with automation. |
|
Lack of AI Expertise | AI governance isn’t just an IT issue—it requires company-wide awareness. A survey by Gartner found that 56% of organizations lack AI expertise. If employees don’t understand AI risks, governance won’t stick. |
|
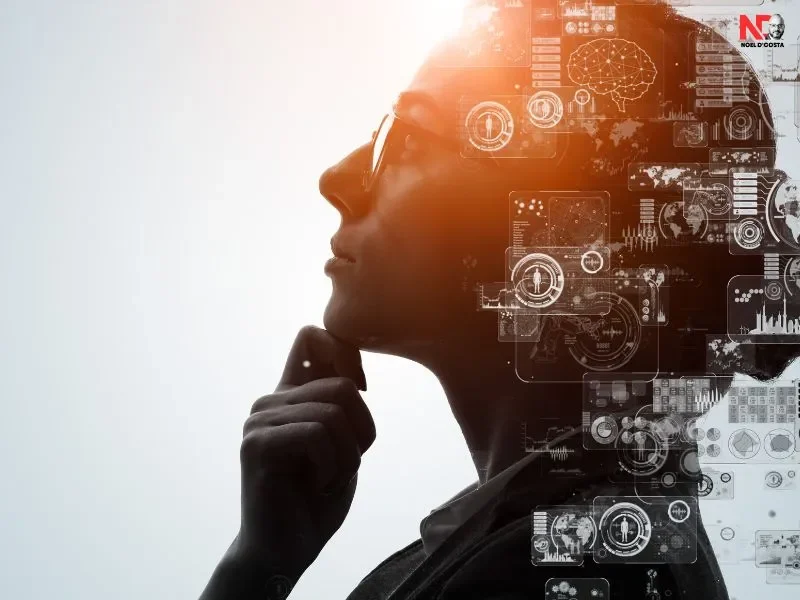
Future of AI Governance: Trends & Emerging Regulations
AI governance isn’t static—it’s evolving as fast as the technology itself. Governments worldwide are scrambling to keep up, drafting new regulations to control AI risks while ensuring its benefits aren’t lost in bureaucracy. The EU AI Act is leading the charge with a risk-based approach, categorizing AI applications by their potential harm. Meanwhile, the U.S. AI Bill of Rights focuses on privacy, bias reduction, and transparency. If companies ignore these shifts, they’ll struggle with compliance when these regulations become enforceable.
Global AI Regulations: What’s Changing?
The EU AI Act introduces strict prohibitions on high-risk AI, including real-time facial recognition and biometric tracking. In the U.S., federal agencies are rolling out sector-specific AI policies, and the FTC is aggressively enforcing AI transparency rules. China has taken a different route, requiring algorithmic audits and government registration for AI-driven services.
- Actionable Step: Businesses operating globally need an AI compliance roadmap that adapts to multiple regulatory frameworks.
- Actionable Step: Legal and tech teams should collaborate to ensure AI models meet jurisdictional requirements before deployment.
AI Governance for Generative AI & LLMs
Large Language Models (LLMs) and Generative AI have raised new ethical concerns. AI-generated misinformation, deepfakes, and copyright infringement are top regulatory priorities. Governments are pushing for content provenance tracking, requiring AI-generated content to be tagged and traceable.
- Actionable Step: Organizations deploying Generative AI should implement watermarking and audit trails to track AI-generated outputs.
- Actionable Step: Developers must ensure bias and toxicity testing is built into LLM governance.
Evolving AI Risk Assessment Methodologies
Regulators are introducing new AI risk assessment models that go beyond traditional cybersecurity frameworks. AI now requires continuous risk audits, bias detection protocols, and explainability metrics.
- Actionable Step: Businesses should integrate automated AI risk monitoring instead of relying on periodic audits.
- Actionable Step: AI governance teams should regularly update risk thresholds and compliance measures based on evolving best practices.
AI governance isn’t just a legal issue—it’s a business survival strategy. Companies that take proactive steps now will avoid compliance headaches later.
Future of AI Governance: Trends & Emerging Regulations
Category | Description | Actionable Steps |
---|---|---|
Global AI Regulations | The EU AI Act introduces strict prohibitions on high-risk AI, including real-time facial recognition and biometric tracking. In the U.S., federal agencies are rolling out sector-specific AI policies, and the FTC is aggressively enforcing AI transparency rules. China has taken a different route, requiring algorithmic audits and government registration for AI-driven services. |
|
AI Governance for Generative AI & LLMs | Large Language Models (LLMs) and Generative AI raise new ethical concerns. AI-generated misinformation, deepfakes, and copyright infringement are top regulatory priorities. Governments are pushing for content provenance tracking, requiring AI-generated content to be tagged and traceable. |
|
Evolving AI Risk Assessment Methodologies | Regulators are introducing AI risk assessment models that go beyond traditional cybersecurity frameworks. AI now requires continuous risk audits, bias detection protocols, and explainability metrics. |
|
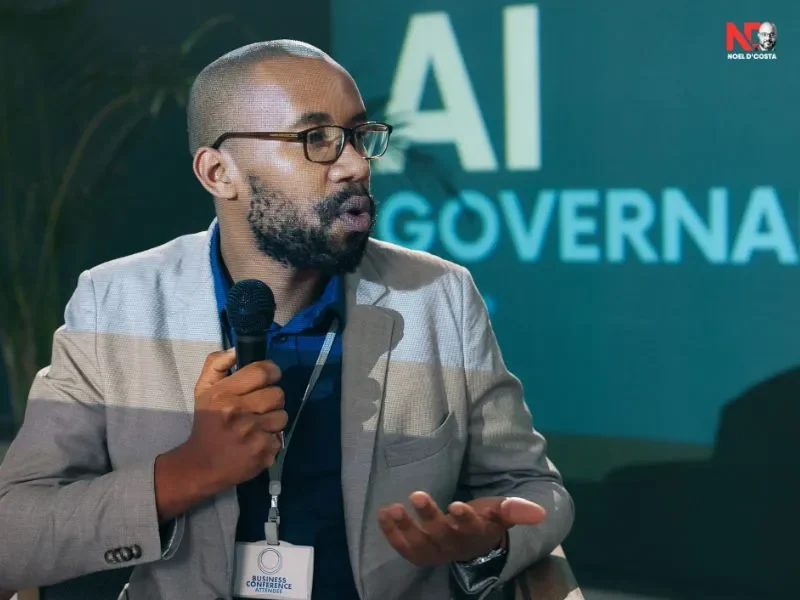
Conclusion
AI governance isn’t just another compliance task—it’s the backbone of responsible AI adoption. Companies that ignore it open themselves to legal risks, ethical failures, and loss of public trust. The numbers tell the story: 81% of consumers say they need to trust a company before buying from them, according to Edelman’s 2022 Trust Barometer. AI decisions impact hiring, lending, healthcare, and criminal justice. If businesses don’t establish clear governance, AI can reinforce bias, make unchecked decisions, and create liabilities.
Where Do You Start?
A strong AI governance framework starts with defining principles. What role does AI play in your business? How will you ensure fairness and accountability? Companies like Google and Microsoft have set up AI ethics boards to guide decision-making. You don’t need a massive task force, but you do need clear policies.
- Step 1: Align AI governance with your business and legal strategy.
- Step 2: Set up internal AI risk assessments to flag potential compliance gaps.
- Step 3: Train employees—AI isn’t just an IT issue; it’s a company-wide responsibility.
Why It Matters Now
Regulations are moving faster than most companies expect. The EU AI Act is set to impose strict penalties for non-compliance, and the U.S. is rolling out its own AI Bill of Rights. Businesses that wait will face costly legal and operational disruptions.
Start now. Build your AI governance framework before external regulators force your hand. It’s not about avoiding penalties—it’s about ensuring AI works for your business, not against it.
Frequently Asked Questions
1. What is an AI Governance Framework?
An AI Governance Framework is a structured approach that organizations use to manage AI-related risks, enforce compliance, and ensure transparency, security, and accountability. It defines policies, ethical guidelines, and regulatory compliance measures that guide AI-driven decision-making.
Without a governance framework, AI systems can become unpredictable, leading to biased outcomes, security vulnerabilities, and legal challenges.
2. Why is AI governance important in SAP implementations?
AI is increasingly embedded in SAP implementations, automating financial processes, supply chain management, HR decisions, and more. While this increases efficiency, poor governance can introduce risks such as non-compliance with data privacy laws, security breaches, and biased decision-making in hiring or credit scoring.
A governance framework ensures that AI operates ethically and aligns with business objectives while minimizing legal and operational risks.
3. How does AI governance help with regulatory compliance?
Regulations like GDPR, the AI Act, ISO 42001, and NIST AI RMF require organizations to ensure AI systems are transparent, fair, and secure. Without governance, businesses risk massive fines, legal disputes, and reputational damage.
AI governance establishes protocols for data protection, explainability, accountability, and continuous monitoring to keep organizations compliant.
4. What are the key components of an AI Governance Framework?
A comprehensive AI Governance Framework includes:
- Risk Identification & Mitigation: Detecting and addressing AI risks such as model drift, bias, and data security threats.
- Bias & Fairness Monitoring: Ensuring AI decisions do not reinforce discrimination in hiring, lending, or customer profiling.
- Security & Compliance Controls: Protecting AI systems against adversarial attacks, data poisoning, and unauthorized access.
- Human Oversight & Explainability: Keeping humans involved in AI decision-making and ensuring outputs are understandable.
- Incident Response & Auditing: Establishing protocols for handling AI failures and maintaining audit trails for accountability.
5. How can companies monitor AI risks in real time?
Organizations use automated dashboards, anomaly detection systems, and AI model audits to track risks before they escalate. Real-time monitoring can detect performance degradation, biased decision-making, or security vulnerabilities, allowing businesses to take corrective action before AI-related failures impact operations.
For example, banks use AI fraud detection systems that instantly flag suspicious transactions, preventing financial losses.
6. Who is responsible for AI governance in an organization?
AI governance is not just the responsibility of IT teams—it requires a cross-functional approach. Key stakeholders include:
- Compliance Officers: Ensure AI meets regulatory and ethical guidelines.
- IT & Security Teams: Implement security measures and monitor AI performance.
- Data Scientists & AI Engineers: Develop and audit AI models for fairness and accuracy.
- Risk & Legal Teams: Assess legal exposure and manage AI-related liabilities.
- Executives & Board Members: Oversee AI strategy and align it with business goals.
7. What happens if AI governance is ignored?
Companies that neglect AI governance expose themselves to financial losses, legal penalties, security breaches, and loss of public trust. For example, Amazon had to scrap its AI hiring tool after it was found to discriminate against female candidates, a problem that could have been prevented with proper governance.
In another case, AI-driven trading errors caused a $440 million loss in minutes due to a lack of oversight. Without governance, AI can become a liability rather than an asset.
8. How can businesses implement an AI Governance Framework?
To establish a strong AI governance framework, organizations should:
- Develop clear AI policies and ethical guidelines based on industry regulations.
- Conduct risk assessments and compliance audits to identify vulnerabilities.
- Implement monitoring tools to track AI decisions and flag anomalies in real time.
- Enforce human oversight in critical AI-driven decisions.
- Provide training for employees to understand AI risks and compliance requirements.
- Establish an AI Ethics & Risk Committee to oversee governance and ensure accountability.
9. What is AI Governance?
When large organizations use AI, the risks multiply—one bad decision can impact millions of people. That’s why enterprises need AI governance strategies that:
- Set company-wide AI policies for ethical usage.
- Automate risk monitoring to catch compliance violations early.
- Assign clear responsibilities for AI oversight across departments.
Big companies can’t afford AI failures, so governance helps them scale AI responsibly while keeping regulators and stakeholders happy.
10. What is AI Governance Certification?
If you’re working with AI, certifications can help prove you’re doing it responsibly. Certifications like ISO 42001, NIST AI RMF, and the Certified AI Governance Professional (CAIGP) show that a company or individual knows how to manage AI risks and stay compliant. With AI regulations tightening worldwide, businesses are increasingly requiring governance certifications to avoid fines and reputational damage.
11. What do you need to do to get an AI Governance Job?
AI governance jobs are on the rise because every company using AI needs experts to manage risks. Some of the most in-demand roles include:
- AI Ethics Officer: Makes sure AI decisions are fair and unbiased.
- AI Compliance Manager: Ensures AI follows laws like GDPR and the AI Act.
- AI Risk Analyst: Identifies risks and figures out how to fix them.
- AI Governance Consultant: Advises businesses on AI policy, compliance, and risk management.
As AI regulations expand, demand for these roles is only growing.
12. What is an AI Governance Platform?
AI governance platforms help businesses manage AI accountability without the headache. They provide tools for:
- Bias detection (so AI doesn’t discriminate).
- Explainability reports (so AI decisions make sense).
- Regulatory tracking (so you don’t get hit with fines).
Platforms like IBM AI OpenScale, Fiddler AI, and Microsoft AI Governance Framework help businesses stay compliant and keep AI in check.
13. What are AI Governance Tools?
Think of AI governance tools as your AI watchdogs. They track, audit, and monitor AI systems to spot biases, security risks, and compliance issues before they become big problems. Some popular tools include:
- Google Model Card Toolkit (for transparency).
- Fiddler AI (for fairness and bias detection).
- IBM AI OpenScale (for tracking AI decisions in real time).
These tools help businesses keep AI under control while proving compliance to regulators.
14. What is AI Data Governance?
AI is only as good as the data it learns from. If the data is biased, the AI will be biased. If the data is flawed, the AI will make mistakes. AI data governance is all about keeping data clean, accurate, and compliant. This means:
- Checking for bias before AI models are trained.
- Encrypting and anonymizing sensitive data to protect privacy.
- Following laws like GDPR and CCPA to avoid legal trouble.
Without strong AI data governance, businesses risk security breaches, bad predictions, and lawsuits.
15. What is Enterprise AI Governance?
When large organizations use AI, the risks multiply—one bad decision can impact millions of people. That’s why enterprises need AI governance strategies that:
- Set company-wide AI policies for ethical usage.
- Automate risk monitoring to catch compliance violations early.
- Assign clear responsibilities for AI oversight across departments.
Big companies can’t afford AI failures, so governance helps them scale AI responsibly while keeping regulators and stakeholders happy.
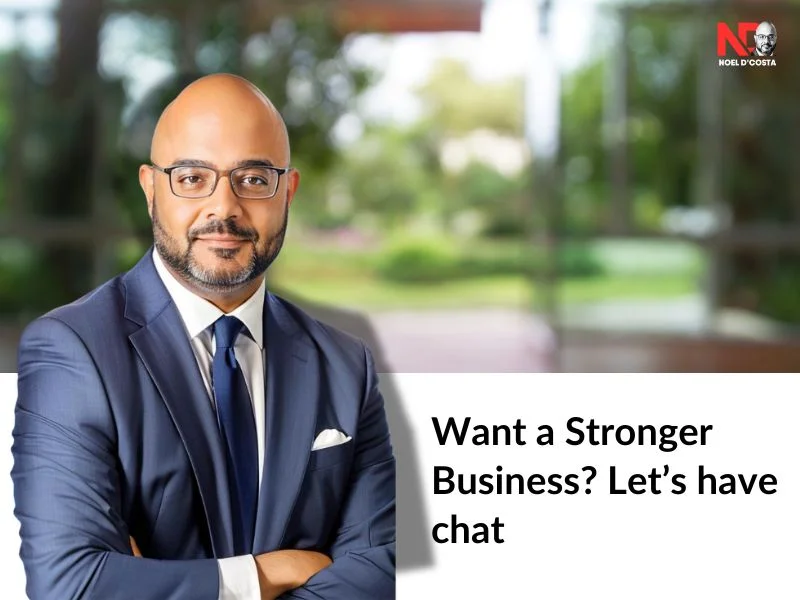
2 Responses